The Guide to Using Artificial Intelligence
Artificial intelligence (AI)—once known as a repeat offender for being long on promise and short on results—has at last become a real, useful technology that is making its way into a wide variety of devices, applications, and services. Banking, manufacturing, medicine, and other industries, along with most branches of the sciences and even the arts, are deploying innovative AI solutions to problems that are difficult or impossible for conventional computer programs to solve.
All of this activity has inspired businesses around the world to incorporate AI technologies into both internal systems and customer-facing products and services. For some companies, AI is a core component of their digital transformation initiatives.
In this article, we describe some of the concepts of AI, what it can be useful for, and how to go about implementing AI into your company’s digital life.
In this guide, we'll break down a normally complex topic into a few segments and identify key components to implementing AI in the real world.
- A definition of Artificial Intelligence?
- Identify the real life limitations of AI
- Illustrate AI's business benefits
- Provide insight to AI successes in business
- Implementing AI in your digital products
- Anticipate the future of AI
- Execution of your AI implementation
DOWNLOAD THIS AI GUIDE
What is Artificial Intelligence?
The broad definition of AI is any computing technology that imitates, or attempts to imitate, human cognitive processing for a specific task or set of tasks. For much of its history, AI was little more than an academic curiosity; research would see boom-and-bust cycles, with long stretches of little progress punctuated by frenzied activity fueled by irrational hype and unrealistic expectations. Only in the last few years has real progress been made in AI research, and many practical systems incorporating the technology are now available or in development.
Modern AI systems fall into the category of artificial neural networks (ANNs) and a subset of ANNs that includes machine learning systems.
An ANN is an arrangement of “nodes” modeled in software, with each node vaguely analogous to a neuron in a human brain. Each node is connected to other nodes in a specific pattern, and each node takes an incoming numerical “signal” from another node, processes it using a mathematical formula, and passes it on to one or more other nodes according to defined rules. The number of nodes, the connections among them, and the formulas and rules are all set according to the task the system is designed to address.
An ANN system takes input data (such as a photo of a moose) and produces an output (such as classifying the object in the photo as “moose” or “not moose”). The ANN must be “trained” to perform the task it was designed for. This means providing huge quantities of input data (in this example, thousands of pre-annotated photos, some containing moose and others not containing moose) so that it learns to recognize patterns in the data that indicate the presence of a moose. Training involves many iterations of processing, evaluation, and tweaking the design parameters to improve the accuracy of the system.
In a machine learning system, the parameters are tweaked automatically. These systems still require large quantities of training data, but they can teach themselves much faster than a designer teach by hand.
Once an ANN system has been trained, it must be tested using input data it has not seen in training, to determine if it has learned its lesson well. If it hasn’t, it’s time for more training, or perhaps a redesign from the ground up.
AI Systems in Action
Many industries and academic pursuits have started to deploy exciting applications powered by AI:
- Science: Finding planets orbiting distant suns does not involve astronomers gazing through telescopes on starry nights. Even the most powerful Earth-bound telescopes are not up to the task. The orbiting Kepler telescope, however, was designed for just that purpose, and AI systems have been combing through the data Kepler sends to locate and characterize exoplanets.
- Medicine: Medical imaging is undergoing an AI-fueled renaissance. AI systems are helping doctors diagnose patients by “seeing” subtle clues in X-rays, MRIs, retinal photographs, and CT scans that indicate the possible presence of disease. This approach helps prevent unnecessary invasive surgeries and biopsies.
- Insurance: While the insurance industry is seldom thought of as forward-thinking, you'd be surprised at the investment placed by insurance companies towards advanced tech. These technologies assist with claims processing, fraud prevention and product offerings.
- Retail and Entertainment: Amazon, Netflix, and other providers can recommend products, movies, and services according to your buying and viewing history. There is always something new and interesting to buy, read, view, or do, and these providers and their AI-powered recommendation systems just might know your tastes better than you do.
- Finance: From ATMs and mobile apps that read handwritten checks to systems that instantly detect and stop fraudulent transactions, AI has become an important tool in financial technology (“fintech”, for short).
- Art: AI systems are being used to compose music and poetry, and to create many types of original visual art pieces.
- Personal assistants: Led by Apple’s Siri, Microsoft’s Cortana, and Amazon’s Alexa, mobile devices and smart home systems are able to understand and execute spoken commands—something that would be almost impossible without AI.
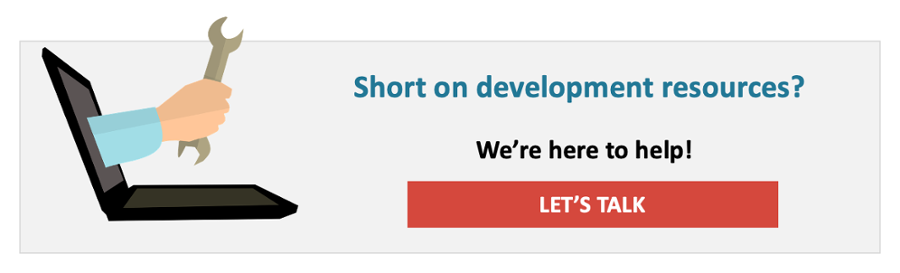
Limitations of AI
At one time it was thought that ANNs would be able to model an entire human brain, enabling a machine to perform any cognitive task. When this dream turned out to be wildly impractical, computer scientists started applying AI to much narrower tasks. Hence the major limitation of artificial intelligence: An AI system can be trained to do one thing (or a few things) very well, but it’s useless for anything else. Google’s champion Go-playing AI system, for example, doesn’t play checkers, or even tic-tac-toe.
AI technology suffers from other limitations as well:
- Designing and optimizing an AI system requires a level of expertise that many developers don’t possess. AI developers tend to command higher prices than developers without that expertise.
- Training and testing an ANN system requires huge amounts of pre-annotated input data. For some applications this data can be accessed free or for a nominal charge, but for specialized applications the data must be annotated by hand, assuming it exists in the first place. If it doesn’t, it must be generated with enough variation for effective training and testing.
- Few AI systems do their jobs with 100% accuracy. Most exhibit some amount of “false positives” and “false negatives.” Depending on the application, this inaccuracy might be acceptable, but in some (such as self-driving automobiles), one false result can be fatal.
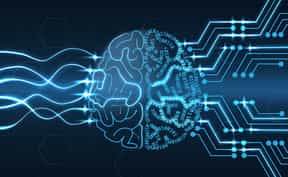
So, if you’re expecting to build or buy an AI system that will be the brains behind a general-purpose Rosie the Robot, complete with snarky attitude, you’re in for some disappointing results. But if you have a narrowly defined task that involves recognizing a small set of patterns in data, read on.
AI's Business Benefits
In contrast to some expectations that AI applications in business will universally provide revolutionary benefits, the reality of AI solutions is much more incremental.
In fact, the relatively brief history of AI is littered with dead ends, solutions that didn’t deliver on their intended benefit, and systems that didn’t live up to the hype. Recently, AI technologies have proven useful for certain narrowly defined problems and are quietly making inroads in the enterprise. Said another way, most AI applications in business aren’t terribly visible. In 2014, technology prognosticator Kevin Kelly wrote that the AI is more like a “cheap, reliable, industrial-grade digital “smartners” running behind everything, and almost invisible except when it blinks off.”[i]
It’s important to understand that companies investing in AI are motivated by the technology’s expected benefits. According to the 2019 Deloitte Technology Trends report[ii], these benefits include:
- Optimizing internal operations by automating manual processes
- Optimizing external operations such as customer service, vendor relationship management, and talent acquisition
- Enhancing current products and services by better identifying customer needs and market trends
- Enabling better business decisions informed by algorithms that account for a larger number of variables
- Assisting with product development and design
- Freeing workers to be more creative by taking over tedious and repetitive tasks now performed by humans
In the context of business, these advantages can result in increased revenues, decreased costs, greater security, better regulatory compliance, and overall competitive advantage in the marketplace. That’s why many companies, large and small are making investments in AI technology.
[i] Thomas Davenport, The AI Advantage, The MIT Press, 2018
[ii] Deloitte Insights, Technology Trends 2019, 2019
AI Success Stories
In his 2018 book, The AI Advantage: How to Put the Artificial Intelligence Revolution to Work, author Thomas H. Davenport notes many successful implementations of AI to-date have been isolated “pilot” projects. They address specific business problems rather than enterprise-wide, “boil-the-ocean” initiatives. There are several reasons for this trend:
- AI solution development often requires expertise that many organizations lack. – This means companies either have to build internal AI development teams from scratch or engage with outside developers.
- AI technology is still in its infancy. – Its capabilities are quite limited. A typical AI algorithm does one thing really well, such as identifying whether an image shows a specific object of interest. Asking the same algorithm to make inferences about that object on the basis of other objects in the image is, for now, asking too much. Most AI solutions are best suited for narrowly defined problem spaces with little ambiguity.
- Most businesses are not eager to make significant capital investments in unproven technology. – They prefer to first apply it to “low-hanging fruit” in order to gain experience with and understanding of its capabilities before rolling it out to address more complex, cross-functional problems.
Many of AI’s success stories are unfolding behind the scenes; applications in internal operations, research and development, and other back-office scenarios. Some examples include:
- Adecco staffing agency is using an AI-powered system to prescreen candidates and match them to open positions on the basis. Variables used include skills and geographic locations. The system is able to identify potential candidates that human recruiters might overlook, resulting in a better set of candidates.
- Google, an acknowledged leader in AI research has deployed an AI-powered system to monitor and manage cooling for its large data centers, resulting in significant reductions in energy usage and operational costs.
- Pharma manufacturer Pfizer uses AI for several business challenges, including compound prediction to identify promising formulas for drug therapies. This system can eliminate months of trial-and-error experimentation.
Implementing AI in your digital products
Do You Really Need AI?
If you want to produce an AI-powered product just to be able to say you’re in the AI club, you’re doing it for the wrong reason. Ask yourself if AI is the only viable approach, or if conventional computing techniques can do the job just as well. AI tends to outperform conventional computing when there is variability in the patterns it is asked to detect. If the data—be it image data, audio data, scientific data, or what have you—contains the same exact pattern over and over, then a conventional approach will do just as well or better than an AI system, without the added cost.
For those cases where it’s difficult to see a clear advantage either way, consider the costs and benefits of each one in making your technology decision. Be sure to factor in the availability of data for training and testing, as well as the added costs of obtaining or generating and annotating sufficient training and testing data. These costs can be significant if pre-annotated data does not already exist in sufficient quantities.
Last, consider the tolerance for error. If less than 100% accuracy is good enough, then an AI system might be a good fit. If not, your solution design should not let the AI system make the final decision, and instead should bring a human into the loop after the AI does the initial analysis.
Draw Hard Boundaries Around the Problem
If you’ve done your due diligence and found that AI is still the right choice, your next task is to define the scope of the solution. This is important because it can mean the difference between on-time, in-budget delivery and a project that blows the budget and timelines out of the water.
For your first AI project in particular, one key to success is observing strict boundaries around the problem to be solved. Business people have a natural tendency to want to expand the scope of a solution to include neighboring problem spaces, in order to solve additional problems that are almost, but not quite, identical to the one that inspired the project in the first place.
Remind them that AI works best with a narrow, focused problem definition. Unlike conventional approaches, where the scope can sometimes be expanded at an incremental cost increase, in most cases doubling the scope of an AI solution will more than double its cost.
Design, Build, Train, Test, Repeat
With the problem and its requirements well defined, it’s time to design and build the AI-based solution. The specific type of AI will depend on the type of problem. There are many types of ANNs, and some are more suited to certain tasks than others. A competent AI developer will know which type to choose.
When the design is complete, the next step is training and testing. This involves not only large volumes of data, but significant computing power, and is often performed with cloud computing resources.
If all goes well with the training and testing, and the application or device that leverages the AI component is ready, you can unleash your creation in production. If not, the design may require some rethinking, and the whole cycle starts over again.
Bring In Outside Experts
If you don’t already have in-house AI development expertise, in most cases you are better off bringing in consultants rather than trying to hire a full-time AI developer. Once you have an AI project with outside consultants under your belt, you can evaluate whether your future AI projects warrant the cost of a staff AI developer, and you will have a better idea of what to look for in an AI resource.
At AndPlus, we have a wide range of AI expertise on our development staff, and we know what it takes to make your AI projects succeed. From choosing the right AI approach to designing the algorithm and training and testing the system, we your full-service AI resource.
The Future of Artificial Intelligence
If you show a human child a single picture of a moose, she will, with no further instruction, be able to identify moose in other pictures, even in simple line drawings or stylized cartoon characters. AI, in contrast, requires thousands of different pictures of moose to get a vague idea of what a moose looks like. This is one of the shortcomings that holds AI back: the long, expensive training and testing phase.
Researchers are hard at work developing AI systems that achieve the same level of accuracy as their current counterparts with less training. If they succeed, it will open up whole new application areas for AI, in particular, those that lack the large volume of training data now required. With shorter, less expensive development cycles, AI will make its way into a much wider range of applications.
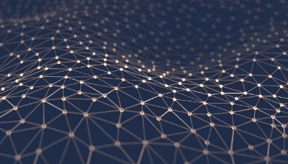
At that point, there will be no limit to what AI can do for a company and its products and services. Now is the time to think about what AI can do for your business, and AndPlus is here to help.
AndPlus And Artificial Intelligence
WORKING WITH A "DIGITAL SHERPA" FOR YOUR JOURNEY INTO ADVANCED TECHNOLOGIES
With proper, thorough planning, and the right guide to build and take you through an AI Roadmap, a project can go flawlessly, or nearly so. Be aware: You might have only one shot to get it right. A failed adoption of an advanced technology can lead to countless unforeseen consequences as you scale.
AndPlus can be your digital Sherpa. We've done it, we’ve seen it all, and we know how to do it right.
Need a quick crash-course in the 'MVP' methodology?
A Minimum Viable Product (MVP) has only those features needed to validate its continued development. Its primary goal is to obtain this insight at a lower cost than that needed to develop a product with more features.
Feature Prioritization
Our process begins by identifying the primary goal that will address both our client's business goals and the end user's goals. We select the methods that the MVP will use to accomplish these goals. Our design team then defines the minimum scope of work and use this list of features to map the ideal user journey.
Early product prototypes are often developed at this stage in order to illustrate concepts and ensure that business objectives and user experiences are aligned and optimized.
Development
Once the user journey is mapped, the code starts flowing. Early prototypes/wireframes are brought to life by our engineering team. We use an Agile Scrum process that is custom tailored to our industry. And that's the kicker. We don't just utilize this same Agile framework straight from the textbook, we optimize the development process based upon more than a decade of development experience gained from hundreds of digital development projects.
Output
The fun part! Our sprints run in 2-week increments. You get actual working builds of your product every two weeks. These builds are tested, and iterated upon as the project moves forward. We pride ourselves on iterating these builds to perfection by launch day.
Our deep expertise and custom Agile development process enable AndPlus to iterate quickly, provide transparency, and deliver on time and on budget — helping our clients get to market faster.